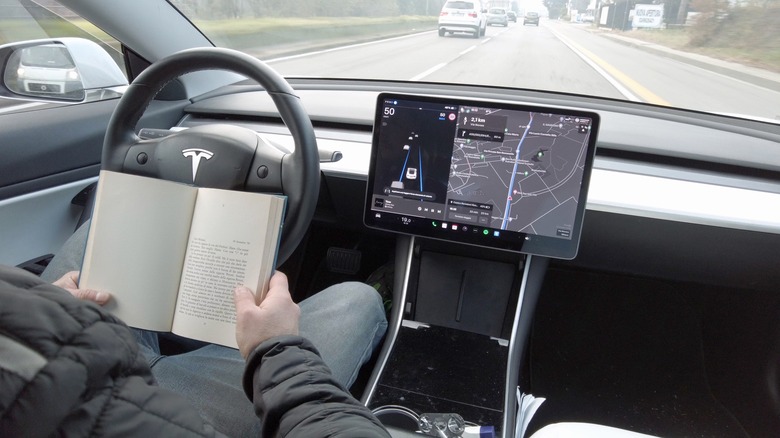
DELBO ANDREA/Shutterstock
The automobile industry is currently moving in the direction of higher autonomy levels, with the ultimate goal of letting a car move around on its own without requiring any human intervention. We’re not quite there yet, even though the likes of Elon Musk have claimed that the existing advanced driver assistance system on Tesla cars was «probably» better than humans all the way back in 2016. Regulatory bodies have been on the fence about greenlighting ambitious self-driving tech, not because of scientific skepticism but due to a track record of accidents that have resulted in several people losing their lives and instances of self-driving vehicles blocking emergency responders.
The solution, however, could be to reimagine how we train these self-driving systems. «Manufacturers are gathering data on how an autonomous system should be driving from millions of people on the road, but is that really the most authoritative source?» asks Dr. Laine Mears, Automotive Manufacturing Chair at Clemson University’s International Center for Automotive Research. The concerns are valid. Take, for example, Tesla, which relies on audio-visual data from billions of miles driven by millions of Tesla cars currently on the road.
Then there is Shadow Mode, which Tesla describes as «a dormant logging-only mode which lets us validate the performance of the feature in the background based on millions of miles of real-world driving.» But that doesn’t cover edge scenarios, or to put it more specifically, low-frequency, unprecedented rare events, one where human decision-making could be the difference between life and death.
Rules may not be enough for self-driving cars
Gorodenkoff/Shutterstock
The likes of Tesla try to address those unprecedented scenarios with a system called detectors, which learns from visual data collected from a car and then it is beamed back as an iteration. However, despite all those efforts and domain-leading computing prowess, Tesla cars are also involved in the highest number of accidents with driver-assist systems enabled. Mears thinks carmakers should also look at a few other aspects instead of just simulation modeling and training on driver data.
«OEMs have recently transitioned away from rule-based systems, but if we don’t respect the rules of the road absolutely (i.e., laws and regulations), what kind of driving system would result and how effective and predictable could it be?,» Mears tells SlashGear. It’s a solid argument and one that echoes Tesla’s own policies. The company notes that drivers should always be ready to take over the steering wheel, irrespective of whether the self-driving system is engaged or not.
The problem, according to Mears, lies in how AI acquires its decision-making skills. «AI can learn through observation, but it needs to have a clear understanding of right and wrong in order to make the best decisions, like a person,» he tells us. Multiple research papers have highlighted similar risks from «unknown unknowns» and how a shift in training strategy is necessary. «Higher-level AVs require a self-driving system that incorporates a decision-making ability capable of dealing with unknown driving scenes and uncertainty,» says a research paper published in the Sensors journal.
The road ahead for safe self-driving
Gremlin/Getty Images
The road to making a reliable autonomous driving tech stack is not straightforward. Self-driving cars often make short-sighted choices because they don’t fully understand their surroundings and only plan for the very near future. This can lead to mistakes, some of which could be ghastly. Anticipating too far into the future ahead means less accurate predictions, while focusing only on what’s close allows for more accurate but very limited planning. Humans are good at balanced anticipation, allowing them to react more accurately.
Some experts are proposing a fusion model, where autonomous vehicles moving on the road communicate with each other as well as the person behind the wheel for the safest experience at tasks like lane change, overtaking, and entering a highway, among others. Experts at Cornell University recently detailed a memory-based learning system for autonomous vehicles that would use past events as a learning model for the future, while researchers at Korea’s Incheon National University are pushing for an IoT-enabled end-to-end system for 3D object detection in real-time for self-driving vehicles.
Other research proposes a rules-based learning model for autonomous cars along the lines proposed by Mears. However, incorporating a decision-making ability that can handle the unknown, unexpected variables would need more than just a smart AI, which itself would require a lot more data with a focus on dynamic environments. Another radical idea proposed by MIT experts involves remote human supervision for risky scenarios, but scaling and deploying it reliably for millions of cars would be a mammoth challenge on its own.